Key takeaways:
- Multi-domain simulation models exist at many different levels of abstraction based on the decisions product leaders are making at different stages of the product lifecycle. Keeping the model abstractions coherent with each other is the next innovation needed to assure best decisions can be made in the context of current system performance requirements.
- Pervasive sensing enables massive data collection for product performance analytics. Product usage is measured in near real-time providing valuable insights to designers, engineers, and their management.
- Orchestrating the “as used” product measurements and the corresponding simulation models (i.e., digital twins) as decisions are made improves a products robustness as it is designed.
- Investigating customer complaints improves with coordinated models and measurements and will improve robustness for products already in use at customers.
- Altair’s expanding system modeling management combined with their machine learning initiatives to apply measurements to models will advance robust product designs across engineering disciplines: structural, thermal, EMI/EMC, optimization, and controls.
Product Improvements and Complexity Are Increasing
Products are more complex and are changing more quickly. Different functions in a company contribute to product decisions from different points of view using all types of models. MBSE practices can encourage the right decisions at the right time using abstraction models if they are trustworthy. Models are used for earlier product decisions, often based upon models of previous products, but these decisions need confirmation during development as testing measurements become available. Abstracting complexity provides increased transparency through design reviews at any time.[1]
Left unmanaged, the various models can become confusing, contradictory, and counterproductive. Orchestration of trade studies becomes complicated as the product performance envelopes evolve when technology choices are applied to the product being designed. Creation and use of reduced order models using computer-based engineering tools is necessary.
Operational product risks and the related safety margins need to take advantage of all usage insights—even if they occur after a product is delivered. Models should continue to adapt as empirical data is collected. Product upgrades are made more confidently when models represent reality: e.g., usage, multi-physics coupling, and material durability.
Staying integrated from the start enables better collaborative decision making using contextual systems models. Traceability from requirements to products and from products back to requirements improves with contextual models. A tools/methods environment providing appropriate model fidelity is needed using reduced order models adequate for the decisions to be made. Managing the workflow from one decision to the next in an organization as decisions are made is needed, especially as it involves several unique roles, see Figure 1.
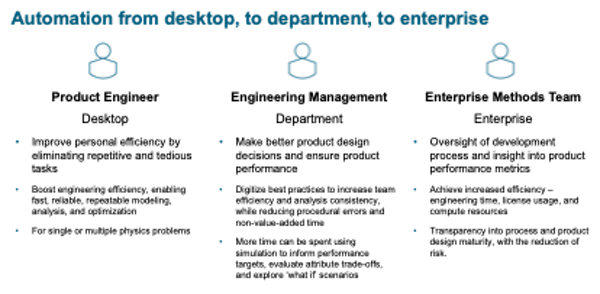
Figure 1—Different Roles Collaborating Need Shared, Trusted Contexts
(Courtesy of Altair)
Meaningful Models at Each Decision Point
Addressing the needs of model users—managers, designers, and release engineers who use models when making decisions—is the next frontier. A model is useful when it helps the user, not just the simulation expert, make their next decision. The order of decisions is driven by company processes which evolve to keep an enterprise competitive. As technology and complexity change, the decision progression changes. The orchestration of models at different fidelities and different maturities at different times is needed to keep models meaningful.
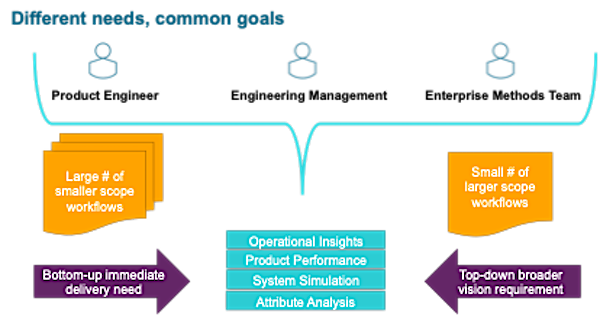
Figure 2—Different Needs, Common Goals
(Courtesy of Altair)
Altair’s Solutions for Meaningful Models with Automation
While Altair is known primarily for being an engineering modeling and simulation company, they have expanded their product scope and customer base significantly over the past decade into complimentary technologies in material data, conceptual design, manufacturability assessment, scalable high-performance computing, big data analytics, and physics-based simulation enhanced via the application of AI/ML technologies. The use of empirical data, analytics and AI/ML will provide business and engineering insights, improving decisions. What makes Altair unique is their focus on concept workflow exploring the functional and performance envelope producing a robust design.
Simulation driven design has been used extensively in automotive and aircraft design using Altair’s modeling and simulation solutions. There are benefits when the physics coupling between vibration, heat, and interferences are seen and resolved together across all physical domains to improve product durability and mass production efficiency. Altair’s CAE capabilities provide benefits from a systems context while gaining more insight from data (predictive (simulation) and measured (empirical))—it is more thorough.
Empirical data tunes digital twins that serve the entire enterprise, including not only product development and engineering functions but also business and financial functions as well as manufacturing and in-service operations. Digital twins are becoming instrumental to product development and operations.[2] Just a few years ago, most engineers identified a single digital twin as a virtual, static assembly or mockup for design reviews. Now they identify many more types of digital twins supporting all kinds of product decisions, many beyond 3D design. These are clear indicators that digital twins are being enhanced to improve decisions. They do this by constantly improving models, making models meaningful just in time.
Examining performance from a consistent user experience with each critical engineering discipline at each decision point encourages discipline specialists to interact and improve product designs before prototype samples are manufactured. It even provides the basis for quality management focused on consumer insights based on customer product usage. Altair realizes the next step is expanding proven CAE/AI/ML techniques across all engineering disciplines at earlier points in product development—the left-hand side of the systems engineering Vee. They summarized their vision for Requirements to Production as shown in Figure 3.
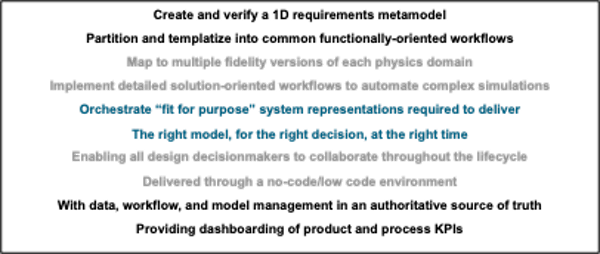
Figure 3—Vision for Requirements to Production
(Courtesy of Altair)
Removing the administrative work to organize and abstract models, improve them when empirical measurements are received (which is happening more frequently, enabled by IoT), and adjusting decision processes (the order and degree) are the catalyst behind Altair’s solutions. Altair has many core modules including Inspire, Pulse, and Knowledge Studio. Pulse orchestrates better attribute analysis. Activate manages system simulation. Product performance assessments are managed by Altair One. Knowledge Studio manages measurement (empirical) data. They work in concert utilizing a data platform. When assembled they make an application which is focused on the key decisions using trustworthy models. As more empirical data is acquired, the same application encourages exploration of the limits of a design, improving design optimization and risk management.
Altair’s entire spectrum of tools and solutions provides the basis for learning models—meaningful to the next decision. Figure 4 summarizes Altair’s Battery Designer and its use of their core modules: Pulse, SimLab, HyperWorks, and many more.
Figure 4—Altair Core Modules Provide Building Blocks Enabling Meaningful Models
(Courtesy of Altair)
Product Improvements During Operations
Insights from empirical data are always used by successful enterprises. Many products have histories of small incremental improvements where the manufacturing, service, and use of gained knowledge is basically stable, limited by the ability to survey and consolidate insights. Remote sensing in actual products is speeding the collection of usage data which can be used to improve models making them more trustworthy. Sensing is becoming much more affordable as electronics miniaturization occurs. ML/AI will receive more data to improve learning and simulation models. Sensor placement and Edge computing (done at the sensor) are driving more and more mechatronics designs. Presenting empirical measurements using Altair’s Panopticon makes conveying insights easier. Altair’s multi-domain simulation solutions, Panopticon, and their AI/ML focus with CAE are providing additional decision-making capabilities leading to more robust products.
Customer Testimonial
“We share a common vision on the convergence of AI and engineering to drive significant positive business outcomes. Altair has unique domain expertise and best-in-class, low-code data analytics technology. This collaboration will enable us to bridge the gap between engineering and data science, and empower our engineers to truly be engineers, focused on extracting the benefits of machine learning and AI from our data,” said Dr. Peter Wehle, Head of Innovation and R&T, Rolls-Royce Deutschland. “Ultimately this collaboration will help to democratize our data analytics, enabling our engineers to make better daily data-driven decisions, and transform our business and products.”[3]
Conclusion
Altair understands and fosters the importance of systems simulation driven design and manufacturing. They are expanding their integrated solutions portfolio to address orchestration of models as decisions are made and usage measurements are acquired. This follows their proven journey of mechanical, electronic, and mass production use of models (multi-physics, layout/flow) that Altair has fostered for decades. Altair’s CAE track record and their recent additional focus on data analytics will continue to improve their position to maintain meaningful models throughout a product’s life. The notion of meaningful models—models which enable timely, earlier decisions, provides a mindset to development applications. Key in this development is the ability to share insights and views across disciplines, even after mass production—maybe leading to new revenue streams with service upgrades.
Altair has always had a system of systems view. Sensor miniaturization sets the stage for even more empirical measurements, accelerating data collection leading to improving AI/ML applied to product evolution and upgrades. CIMdata recommends companies consider Altair’s core modules and the application environments they can assemble to further accelerate engineering of products while managing new product development risks. The business challenge is enabling smart people, augmented by the effective use of digital technologies, to achieve the business objectives. Business agility, creativity, and product innovation will always prevail. Altair’s simulation expertise, application of AI/ML, and core modules lead to integrated decision-making environments.
[1] Research for this commentary was partially supported by Altair.
[2] https://www.cimdata.com/en/resources/complimentary-reports-research/commentaries/item/17318-technology-convergence-accelerating-for-a-smarter-more-connected-world-commentary
[3] https://www.altair.com/newsroom/news-releases/altair-and-rolls-royce-germany-join-forces-to-converge-artificial-intelligence-and-engineering/